As data management techniques mature, we've seen corresponding growth in the array of tools and architectures available for securely storing, effectively governing, and thoroughly analyzing enterprise datasets. Over the past several years, those architectural options have coalesced around three commonly encountered styles: Data Warehouses, Data Lakes, and Data Lakehouses. The evolution of data storage and management systems reflects the continuous need to adapt to the growing volume, variety, and velocity of data generated in the modern digital world. While each option has specific use cases, each offers unique benefits and challenges. It can be perplexing to determine the right choice for a given organization. In this article, we will delve into the distinctions among these three options and provide insights on when each option might be the most suitable choice. By understanding the advantages and disadvantages of each, organizations can make an informed decision about which data management solution is best for their needs.
A Short History
In 1988, computer scientist Barry Devlin published "A Seven-Step Methodology for Data Warehouse Design," which revolutionized the role data would play in business. The paper crafted Data Warehousing concepts, including ingesting source data into a centralized data repository and using Data Warehousing techniques to support decision-making.
The idea of a Data Lake originated in the late 2000s in response to the challenges faced by Devlin-style Data Warehouses in dealing with the large and diverse data generated by modern businesses. With the advent of the World Wide Web and the Internet of Things (IoT), enterprises needed to store massive amounts of raw data, including unstructured data such as log files and clickstream data. The Data Lake concept emerged as a solution for storing and processing this big data, using distributed file systems such as Apache Hadoop Distributed File System (HDFS) or cloud-based file systems such as Amazon S3 and Google Cloud Storage. The term "Data Lake" describes the vast repository of raw, unstructured data. Over the years, many tools have been developed to allow the query and manipulation of this type of unstructured data. This has opened up the possibility of storing enormous amounts of data at a relatively low price. In addition, the technology has continued to evolve, providing the ability for ACID transactions in a lake through technology such as Apache Iceberg. Queries have become far more complex using some native cloud tools such as Athena combined with federated queries, Redshift Spectrum, and also using 3rd party tools such as Presto. In addition, transformations have become remarkably easier as frameworks like AWS GLUE and AWS Data Brew provide a simple way to create complex data pipelines orchestrated by workflows.
A Data Lakehouse is a concept that builds on the foundation of a Data Lake but adds a layer of structure and governance to the Data Lake that we see in a Data Warehouse. A Data Lakehouse provides a way to manage the entire data lifecycle, from ingestion to retirement. The Data Lakehouse allows the application of business logic, data validation, and security controls to the data as it is stored in the Data Lake. A Data Lakehouse can also provide a more user-friendly environment for business users to access and analyze the data stored in the Data Lake without navigating complex technical processes. One of the largest players in this space over the last several years has been Databricks. Databricks have brought ACID transactions by releasing a hosted version of Delta Lake. Amazon Web Services have also brought its Data Lakehouse architecture solutions that involve a combination of services to bring some of the same benefits of a Data Lakehouse. See the example below:
Source: https://aws.amazon.com/blogs/big-data/build-a-lake-house-architecture-on-aws/
Wait, a Data Lakehouse sounds a lot like a Data Warehouse.
A Data Lakehouse is a hybrid between a Data Lake and a Data Warehouse. It is designed to store both structured and unstructured data in its raw format, allowing a greater variety of data types to be stored. However, it also includes a Data Warehouse-like feature set, making it easier to query the data and perform aggregations. This allows organizations to store their data flexibly and evolve as their needs change. In addition, there can be massive cost savings when considering the difference between an always-on Data Warehouse and a Data Lakehouse.
Can’t I just use a Data Warehouse?
Maybe. As storage costs continue to plummet, many opt for solutions that skip the Lake altogether and ingest right into the Data Warehouse. Data Warehouses are best suited for structured data. They can provide faster query and analysis performance, while Data Lakehouses are better for storing and processing large amounts of unstructured and semi-structured data. While there are similarities, Data Warehouses have a long track record of solid performance against high volumes of data with complex requirements. This does involve extensive data modeling and setup to ensure the data is optimized to be queried in a performant way. A Data Lakehouse wants to be able to provide the queries for structured data but also the unstructured data alike. That convenience comes at the cost of performance and speed of queries.
Data Lakehouse vs Data Lake vs Data Warehouse: Which do I choose?
Choosing between a Data Lake vs Data Warehouse vs Data Lakehouse for your data management and analytics needs ultimately depends on your organization's specific requirements and goals. Each of these solutions has its benefits and trade-offs. The best choice for you may vary depending on the volume and complexity of your data, the need for real-time insights, the level of data governance and security required, and the available resources. It is common to implement multiple solutions to take advantage of their features.
A Data Lake is best suited for organizations with large amounts of unstructured data and, thus, needs to store and process it in its raw form. A Data Lake allows you to store all types of data in any format and at a low cost, providing a flexible and scalable solution for your data storage needs. While storing the data is inexpensive, querying the data can be time intensive, so we often see hot performant data needs in the Data Warehouse in conjunction with using a Data Lake.
A Data Warehouse is ideal for organizations that store large amounts of structured data and also need to provide fast and efficient access to data for reporting and analytics purposes. Data Warehouses are optimized for querying and aggregating data stored in use case-aligned schemas, making them a good choice for organizations that need real-time insights and require high performance and security. There are additional downsides to a Data Warehouse, including cost. Also, data must be structured, which can require expensive munging to ensure the data is properly structured for high-speed performance. This also is evolving as Data Warehouses are now developing the ability to read and query data directly from a Data Lake. Redshift Spectrum, as well as Snowflake, provide this functionality.
A Data Lakehouse is a hybrid solution that combines the benefits of a Data Lake and a Data Warehouse, allowing you to store raw and structured data in one place and providing a centralized platform for data management and analytics. There are still some downsides to using a Data Lakehouse. Query speed is often not as performant as a traditional Data Warehouse. While speeds are getting better, we often see “hot” data placed in a Data Warehouse to provide the level of required performance.
As a seasoned professional services firm in data architecture, Unicon is ready to assist your organization in selecting the optimal data management solution that aligns with your institution's unique needs. Our team is eager to converse with you and share our comprehensive approach to help you make informed decisions.
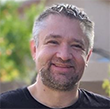