Over the last two decades, organizations have collected staggering amounts of data and are now moving on from asking “Do we have all the data we need?” to “How can we manage and leverage this data to serve our needs?” To navigate these modern data challenges, organizations are finding the need for data governance. Data governance provides direction and clarity amidst these complexities. It forms the foundation of every decision, secures every transaction, and safeguards every piece of information. Data governance is essential for the modern data-informed organization, enhancing data quality, facilitating informed decisions, fortifying data security, and ensuring regulatory compliance.
Data governance systematically organizes, documents, and manages key data assets through defined policies, procedures, and standards. Its structured framework ensures data accuracy, consistency, security, and usability and facilitates control over data access and the maintenance of regulatory compliance. Enhancing data quality in this way provides a reliable basis for analysis and insights that support better decision-making.
Additionally, data governance promotes data security and privacy through rigorous access and usage protocols, a crucial aspect in industries with strict data handling and reporting requirements. The ultimate benefit of effective data governance is improved trust in data, enabling organizations to leverage their data more efficiently, thereby leading to operational efficiency, risk reduction, and competitive advantage.
A brief introduction to data governance
Data governance consists of several core components, each playing a unique role in ensuring the effective management of an organization's data assets. These components include Data Quality, which ensures the accuracy and consistency of data; Data Management, which involves the storage, retrieval, and deletion of data; Data Policies, which outline the rules for data usage and handling; Business Process Management, which aligns data governance with business processes and goals; and Risk Management, which mitigates potential data-related risks and threats.
When delving into data governance, the term "domain" often takes center stage. A domain is a specific area of an organization's data landscape that shares common attributes, characteristics, or functionalities and requires coordinated management. The domain could be defined around a specific business function, data type, or subject area such as customer data, financial data, employee data, or product data. Governance domains facilitate efficient data governance by allowing organizations to group related data, prioritize their efforts, and effectively allocate resources.
Key roles in the data lifecycle
A data steward is a critical role within an organization responsible for maintaining and enhancing the value of data assets. This person manages data sources and ensures their quality, accessibility, and security, all while adhering to applicable legal, ethical, and regulatory standards. Their duties often encompass establishing and implementing data standards, governance policies, and procedures, including data definitions, taxonomies, and metadata management. They also have to ensure data integrity and accuracy, troubleshoot data issues, and provide guidance on the use and interpretation of data.
Key stakeholders have distinct roles and responsibilities in a data governance framework. Data Owners are the personnel who have administrative control and responsibility for data in their respective domains. Data Users are the end-users who need access to high-quality, reliable data for their everyday tasks. IT teams provide technical support to implement and maintain data governance initiatives.
The role of technology in data governance must also be considered. Various tools, platforms, and software facilitate data governance processes. These technologies aid in data cataloging, metadata management, data quality management, and data privacy and security measures. They streamline and automate tasks, providing a more efficient and effective way to manage vast amounts of data and ensuring that data governance policies are applied consistently across the organization. Data governance significantly impacts multiple aspects of an organization's data handling, including regulatory compliance, decision-making, data security, and data quality.
What a successful data governance strategy provides:
Improved Decision-making: Data governance bolsters an environment of data-driven decision-making within organizations. Assuring reliable and valid data availability enables organizations to make informed decisions grounded on precise insights. Moreover, with defined policies and guidelines, data governance ensures uniform interpretation and use of data, thus reducing the potential for decisions based on faulty information.
Regulatory Compliance: Organizations often face stringent data-related regulations and laws such as GDPR. Compliance with these is a top priority, which is where data governance comes into play. It facilitates meeting these requirements by establishing comprehensive data usage, storage, and disposal guidelines. Furthermore, it guarantees the proper documentation and traceability of data handling procedures, a crucial factor during audits or regulatory checks.
Enhanced Data Security: The role of data governance in an organization's data security and privacy is direct and significant. It lays out clear rules on data access, including who can access what data, when, and for which reasons, thereby mitigating the risk of data breaches. It also provides protocols for data encryption, anonymization, and secure disposal, bolstering the organization's data security measures.
Improved Data Quality: Data governance is vital in preserving data accuracy, consistency, and reliability within an organization. It applies data validation, standardization, and deduplication measures, ensuring the data is clean and trustworthy. Furthermore, it fosters a culture of data stewardship, where stakeholders understand and appreciate their role in maintaining data quality.
What are some of the challenges?
Resistance to Change: Like any major organizational initiative, data governance can encounter resistance due to the change it brings to established practices. It's crucial to manage this change effectively to gain stakeholder buy-in. This can be achieved through regular communication about the benefits of data governance, involving stakeholders in the planning and implementation processes, and providing adequate training to ease the transition.
Data Silos: Data silos, where data is kept separate within different departments or units, can hinder the effectiveness of data governance. Breaking down these silos and promoting data sharing is crucial. This can be accomplished by establishing company-wide data policies, fostering a culture of collaboration and data sharing, and employing tools and technologies that facilitate centralized data access and management.
Scalability Issues: As businesses grow, their data governance strategies must scale accordingly. Adopting flexible and robust data governance frameworks and tools that can handle increasing data volumes and complexity is important to ensure scalability. Also, regular reviews and updates to the data governance strategy can help to accommodate changing business needs and data landscapes.
Data governance is a critical aspect of managing the substantial amounts of data that modern organizations deal with daily. It provides direction allowing one to handle inconsistencies and navigate compliance issues confidently. It is not a superficial tool, but a comprehensive framework that enhances an organization's data management capabilities. It directly aids in safeguarding data, enforcing compliance, improving data quality, and enhancing decision-making processes.
Successful governance requires comprehensive planning, cohesive teamwork, strategic implementation, and the integration of cutting-edge technology. These components strengthen the organization's resilience and capacity to manage data effectively.
Where should you begin?
Assessing Current State: The initial step in planning for data governance involves evaluating the existing data management practices. Understanding the current state of data quality, security, compliance, and usage in the organization is crucial. This evaluation helps identify gaps, potential risks, and areas of improvement. It also aids in setting clear and realistic objectives for the data governance initiative.
Building a Data Governance Team: Once the assessment is complete, the next step is to put together a team to carry out the strategy. This involves identifying key stakeholders, typically data owners, stewards, users, and IT teams. Defining the roles and responsibilities of each stakeholder is critical to ensure clarity and accountability in the data governance process.
Developing a Data Governance Framework: With a team in place, the next step is to develop a data governance framework. This framework outlines the policies, procedures, and standards for data usage, management, and security. It also defines the metrics for measuring the effectiveness and success of the initiative.
Prioritizing Data Domains: Not all data is created equal. Some data elements are more critical to the organization's operations and decision-making than others. Therefore, it's essential to identify and prioritize these critical data elements for initial focus in the data governance plan. This prioritization helps manage resources effectively and achieve quick wins, which can support the broader acceptance of the data governance initiative.
Adopting Data Governance Tools: Implementing data governance often requires the support of specific technologies and tools. These may include software for data cataloging, metadata management, data quality management, and data security. Selecting the right tools is crucial as they can significantly enhance the efficiency and effectiveness of data governance efforts.
Implement the Plan: The next step is effectively communicating the data governance plan to all stakeholders, ensuring a shared understanding of the goals, policies, and procedures. It's generally advisable to start with pilot projects in selected data domains that will develop a data product for consumption. These initial projects help solidify the processes and responsibilities of the team members and help identify potential issues or challenges with the new governance processes, and enable the team to make necessary adjustments before expanding the initiative to other domains.
Training and Awareness: For data governance to succeed, all stakeholders must understand their roles and responsibilities. This requires ongoing training and awareness initiatives that ensure everyone knows how to adhere to the data governance policies and procedures. Also, fostering a data stewardship culture can encourage proactive participation in the governance process.
Monitoring and Auditing: Lastly, establishing robust processes for monitoring and auditing is key to maintaining and improving data governance practices. Regular audits can help identify any deviations from the policies, while monitoring can provide ongoing insights into the effectiveness of the governance practices. Both are essential for continual improvement and maintaining high data quality and security standards.
In Summary
This article provides an overview of data governance, highlighting its significance for modern organizations dealing with enormous data volumes daily. Data governance, far from being a superficial tool, forms an integral framework that fortifies data management capabilities by ensuring data quality, facilitating informed decision-making, safeguarding data security, and enforcing regulatory compliance.
Implementing data governance demands a strategic approach to mitigate challenges like resistance to change, data silos, and scalability issues. Effective management coupled with strategic planning helps to overcome these barriers, leading to a seamless transition.
Key steps to implementing a successful data governance strategy include assessing the current state of data management, building a dedicated data governance team, developing a robust data governance framework, and prioritizing data domains. The roll-out plan involves communicating clearly with all stakeholders, adopting data governance tools, and implementing. This phased roll-out helps identify potential issues early and ensures better alignment with organizational objectives.
An essential component of successful data governance is ongoing training and awareness programs for all stakeholders, fostering a culture of proactive participation and data stewardship. Also, regular monitoring and auditing ensure policy adherence, providing insights for continuous improvement of data governance practices.
In essence, data governance is a journey, not a destination. It requires constant evolution in line with changing business needs and data landscapes. Nonetheless, effective data governance ultimately steers the organization toward operational efficiency, risk reduction, and competitive advantage goals, establishing a solid foundation for decision-making and data management.
While the roadmap provided in this article offers a high-level perspective on data governance, it's important to remember that many intricate details require careful consideration. Implementing a data governance plan is a complex process involving numerous moving parts, from understanding your existing data management practices to strategizing an effective roll-out plan and beyond. With our data and analytics services, Unicon can provide the skill and knowledge to guide your organization through these intricacies. We can help you navigate the planning, development, and implementation of a robust data governance solution tailored to your organization's unique needs, ensuring you unlock the full potential of your data assets.
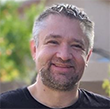