The world is abuzz with chatter about the possibilities of Large Language Model (LLM) Artificial Intelligence (AI) chatbots. Most of us are familiar with chatbots by now. When we approach a service provider for customer support we are greeted by an automated response mechanism. These bots can be very helpful and often produce the answers we need immediately, but these support bots are based on very specific data related to their intended use. LLM chatbots are trained on massive amounts of data (think the entire open internet) and therefore have great flexibility in answering questions and providing service. I have already incorporated the use of several of them in my work routine and that has led me to consider LLM data security and privacy concerns related to their use, as well as what guidelines one should consider when using them. I will address some of those concerns in the rest of this article.
Considerations Regarding LLM Data Security
There are several things to consider when using these relatively new technologies that might not seem entirely obvious to the uninitiated. First is the reality that not everything they tell us is necessarily factual. Yes, they are trained on a massive volume of information and can use that data to deliver useful information, but if some of the training data is inaccurate or if the model through which the AI was trained is flawed, then false information can be the result. It is important to take a position of “trust, but verify” when using the information provided.
Additionally, again based on the nature of the training model used and data supplied, the returned information may be biased in some way. Just as we all carry biases based on our upbringing, education, and life experiences, the data we keep in our minds that causes these biases is not unlike the data that a chatbot is using which may also contain biased assumptions.
Another aspect of these interactions is that the chatbot’s response is entirely guided by the question that it was given (obviously). There have been instances of returned content that was toxic in nature because of the prompt that was given. Sometimes the generation of this content was intentional, but intentional or not it’s vital to keep in mind that our questions, or prompts, that feed into the AI should be carefully constructed and targeted.
Concerns Regarding LLM Data Security
What if, regardless of the potential concerns, the power of these LLM AIs is just too valuable to avoid? How do we use these tools responsibly to not provide them with data that might leak? Fortunately, there are some standard practices to keep in mind that will help.
One of the first things to understand is that as of this writing, the current tools in operation do not include data from your queries into their data set that can then be delivered to other people with similar queries. Current versions don’t automatically insert information from queries into their working data set. However, the information contained in your query is now on the server that hosts the bot and visible to teams that monitor and maintain the service. It’s important to keep in mind that any sensitive data you supply to the bot is now potentially exposed and could be used in training at a later time.
So, what constitutes sensitive data in these cases? It could be actual company proprietary information, or something might be sensitive due to who is asking the question. Consider a company executive seeking perspectives on a pending merger that’s meant to remain confidential. Including health information in the query could reveal something about the asker that should remain discrete. Also, information from different sessions involving the same login could be linked together to reveal more than was intended. It’s important to be thoughtful about what is shared with these services.
How to Stay Safe
The surest way to keep your information private is to use a service that runs locally and has limited internet exposure. This could be feasible for larger organizations, but isn’t realistic for individuals. These systems would still require proper hardening and standard information security protections, but there are fewer concerns with the data that is input as there is greater control over where it goes and who can see it.
Public LLMs are a bigger issue, of course. The best answer is to never include sensitive data in any query. However, to get a specific answer it may be necessary to include information you would rather not and remember that it’s possible for seemingly unrelated information from different sessions by the same individual to be linked and used to extrapolate data that hasn’t been specifically delivered as related. It really boils down to being thoughtful about what you are asking and how you are asking it. Always remember that these LLMs are not your friend and do not have your best interest in mind. As the adage goes, if you aren’t paying for the service, then you are the currency. Your information is the coin of the realm.
Conclusion
LLM AI is a powerful, engaging tool and so useful that it is not likely to go away. The toothpaste is out of the tube and will be difficult, at best, to put back. It is easy to see this capability expanding into every aspect of our lives. So with that in mind, it’s imperative that each individual carefully consider what information to share, how to interpret the results returned and, just as with any sensitive data input into a system, understand the ramifications of that information being exposed as well as the rights claimed in relation to that data by the service provider. Read the privacy statements. Consider the terms of service from the provider and make informed decisions from there. Your privacy and that of your company depend on your diligence.
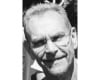